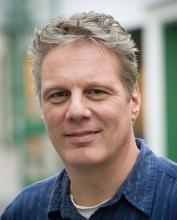
Professor, team leader
+1 (404) 385-7069
CODA building 13th floor
Research Area: Computational Imaging; Machine Learning; PDE-constrained Optimization; Uncertainty Quantification; Randomized Linear Algebra
About me: Felix J. Herrmann graduated from Delft University of Technology in 1992 and received his Ph.D. in engineering physics from that same institution in 1997. After research positions at Stanford University and the Massachusetts Institute of Technology, he became in 2020 faculty at the University of British Columbia. In 2017, he joined the Georgia Institute of technology where he is a Georgia Research Alliance Scholar Chair in Energy, cross-appointed between the Schools of Earth & Atmospheric Sciences, Computational Science & Engineering, and Electrical & Computer Engineering. His cross-disciplinary research program spans several areas of computational imaging including seismic, and more recently, medical imaging. Dr. Herrmann is widely known for tackling challenging problems in the imaging sciences by adapting techniques from randomized linear algebra, PDE-constrained and convex optimization, high-performance computing, machine learning, and uncertainty quantification. Over his career, he has been responsible for several cost-saving innovations in industrial time-lapse seismic data acquisition and wave-equation based imaging. In 2019, he toured the world presenting the SEG Distinguished Lecture "Sometimes it pays to be cheap – Compressive time-lapse seismic data acquisition". In 2020, he was the recipient of the SEG Reginald Fessenden Award for his contributions to seismic data acquisition with compressive sensing. At Georgia Tech, he leads the Seismic Laboratory for Imaging and modeling and he is co-founder/director of the Center for Machine Learning for Seismic (ML4Seismic), designed to foster industrial research partnerships to drive innovations in artificial-intelligence assisted seismic imaging, interpretation, analysis, and time-lapse monitoring.
About me: Felix J. Herrmann graduated from Delft University of Technology in 1992 and received his Ph.D. in engineering physics from that same institution in 1997. After research positions at Stanford University and the Massachusetts Institute of Technology, he became in 2020 faculty at the University of British Columbia. In 2017, he joined the Georgia Institute of technology where he is a Georgia Research Alliance Scholar Chair in Energy, cross-appointed between the Schools of Earth & Atmospheric Sciences, Computational Science & Engineering, and Electrical & Computer Engineering. His cross-disciplinary research program spans several areas of computational imaging including seismic, and more recently, medical imaging. Dr. Herrmann is widely known for tackling challenging problems in the imaging sciences by adapting techniques from randomized linear algebra, PDE-constrained and convex optimization, high-performance computing, machine learning, and uncertainty quantification. Over his career, he has been responsible for several cost-saving innovations in industrial time-lapse seismic data acquisition and wave-equation based imaging. In 2019, he toured the world presenting the SEG Distinguished Lecture "Sometimes it pays to be cheap – Compressive time-lapse seismic data acquisition". In 2020, he was the recipient of the SEG Reginald Fessenden Award for his contributions to seismic data acquisition with compressive sensing. At Georgia Tech, he leads the Seismic Laboratory for Imaging and modeling and he is co-founder/director of the Center for Machine Learning for Seismic (ML4Seismic), designed to foster industrial research partnerships to drive innovations in artificial-intelligence assisted seismic imaging, interpretation, analysis, and time-lapse monitoring.
-
“Advancing Geological Carbon Storage Monitoring with 3D Digital Shadow Technology”, in EAGE Annual Conference Proceedings, 2025. ,
-
“Optimizing CO2 Storage Monitoring with Enhanced Rock Physics Modeling and Optimal Pressure Control”, in CCUS 2025 - Carbon Capture, Utilization, and Storage Conference, 2025. ,
-
“Optimizing CO2 Storage Monitoring with Enhanced Rock Physics Modeling and Optimal Pressure Control”, in CCUS 2025 - Carbon Capture, Utilization, and Storage Conference, 2025. ,
-
“WISER: multimodal variational inference for full-waveform inversion without dimensionality reduction”, Geophysics, vol. 90, 2025. ,
-
“Robust Digital Twin for Geological Carbon Storage”, in SIAM Conference on Computational Science and Engineering (CSE25), 2025. ,
-
“Industry-Scale Uncertainty-Aware Full Waveform Inference with Generative Models”, in SIAM Conference on Computational Science and Engineering (CSE25), 2025. ,
-
“Uncertainty-aware machine-learning enabled velocity-model building with Devito”, in 18th Annual Energy High Performance Computing Conference 2025, RICE, 2025, p. 4th Annual Devito User and Developer Workshop. ,
-
“Digital Twins in the era of generative AI — Application to Geological CO2 Storage”, in ICON Seminar in IoT, 2024. ,