 |
 |
 |
 | Simply denoise: wavefield reconstruction via jittered
undersampling |  |
![[pdf]](icons/pdf.png) |
Next: Favorable recovery conditions
Up: Basics of compressive sampling
Previous: Basics of compressive sampling
Consider the following linear forward model for the
recovery problem
 |
(1) |
where
represents the acquired data,
with
the unaliased signal to
be recovered, i.e., the model, and
the restriction operator that collects the acquired samples from
the model. Assume that
has a sparse representation
in some known transform domain
, equation 1 can now be reformulated as
with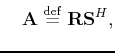 |
(2) |
where the symbol
represents the conjugate transpose. As a
result, the sparsity of
can be used to overcome the
singular nature of
when estimating
from
. After sparsity-promoting inversion, the recovered signal
is given by
with
s.t. |
(3) |
In these expressions, the symbol
represents
estimated quantities and the
norm is defined as
, where
is
the
entry of the vector
.
Minimizing the
norm in equation 3 promotes
sparsity in
and the equality constraint ensures that the
solution honors the acquired data. Among all possible solutions of the
(severely) underdetermined system of linear equations (
) in
equation 2, the optimization problem in equation
3 finds a sparse or, under certain conditions, the sparsest
(Donoho and Huo, 2001) possible solution that explains the data.
 |
 |
 |
 | Simply denoise: wavefield reconstruction via jittered
undersampling |  |
![[pdf]](icons/pdf.png) |
Next: Favorable recovery conditions
Up: Basics of compressive sampling
Previous: Basics of compressive sampling
2007-11-27